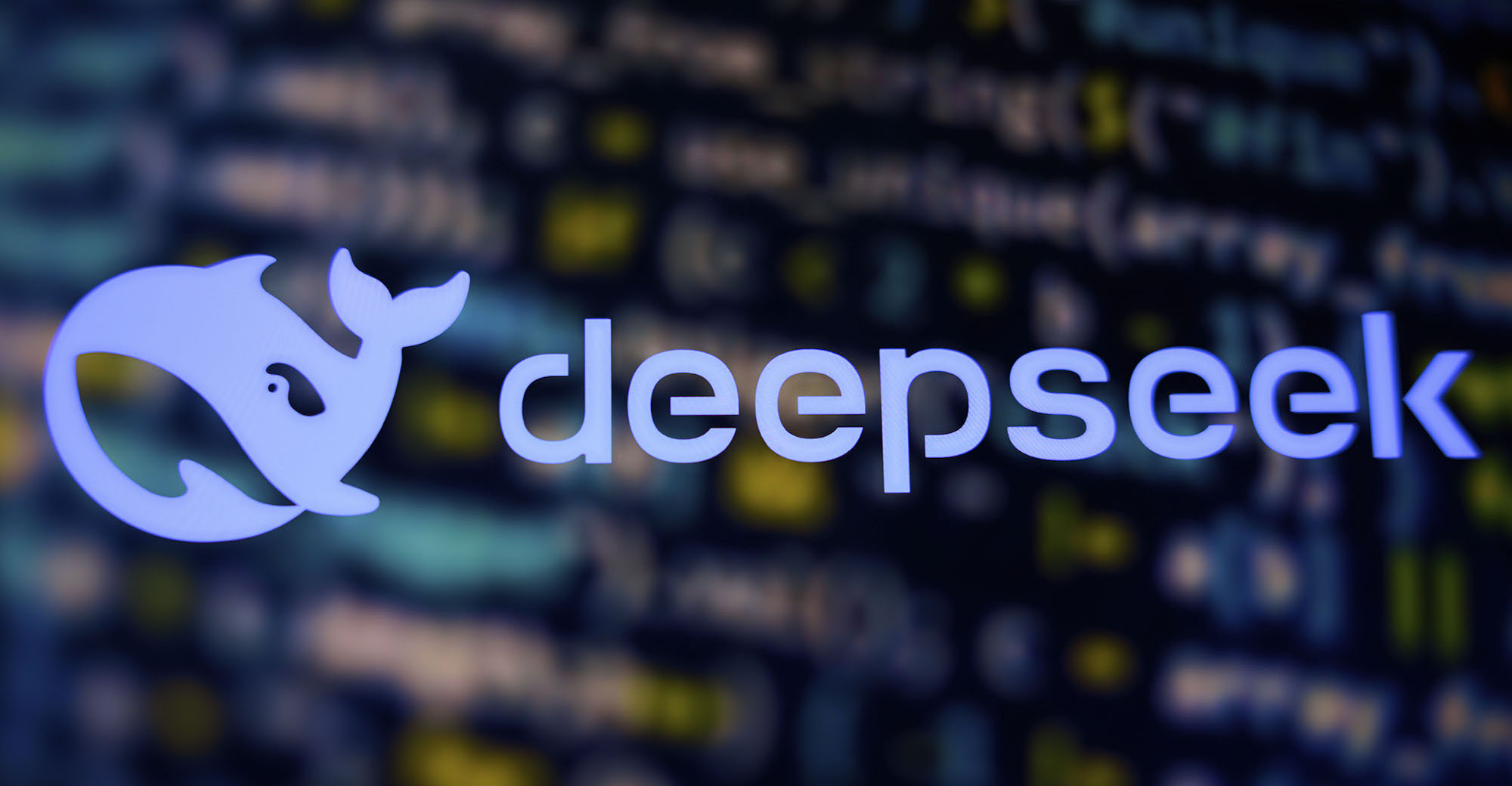
DeepSeek Unveils New Research on Sparse Attention Mechanisms
Want to read in a language you're more familiar with? Please click the pink icon below for one-click AIPlease click the pink icon top right for one-click AI translation
What sets NSA apart is its hardware-aligned design, which ensures optimal utilization of modern computing hardware, enabling ultra-fast training and inference even for long-context tasks.
On Tuesday, DeepSeek released a new research paper titled "Native Sparse Attention: Hardware-Aligned and Natively Trainable Sparse Attention." The paper, co-authored by DeepSeek founder Liang Wenfeng and his team, introduces a technology called NSA (Native Sparse Attention), which could make AI systems faster and more efficient, especially when handling large amounts of data.
Many of today’s AI systems, like those used for language translation or answering questions, rely on something called attention mechanisms. These mechanisms help the AI focus on the most important parts of the information it is processing—like picking out key words in a sentence or important details in a document. However, as the amount of data grows, these systems can become slow and require a lot of computing power, which can be costly and time-consuming.
DeepSeek's NSA addresses these challenges head-on. By introducing a "locally trainable sparse attention mechanism", NSA significantly reduces the computational burden while maintaining high performance. What sets NSA apart is its hardware-aligned design, which ensures optimal utilization of modern computing hardware, enabling ultra-fast training and inference even for long-context tasks. This breakthrough could pave the way for more efficient and scalable AI systems, particularly in applications requiring extensive data processing, such as document analysis, video understanding, and large-scale language modeling.
In simple terms, instead of making the AI analyze every single piece of data in detail, NSA allows the system to focus only on the most relevant parts, skipping over the less important information. Think of it like reading a long article by skimming the headlines and key points instead of reading every word. This approach, called sparse attention, makes the process much faster and less resource-intensive.
Within just two hours of its release, this paper had received nearly 300,000 views, representing a significant advancement in the field of AI, particularly in improving the efficiency of attention mechanisms for large-scale data processing.
SEE ALSO: Chinese City Shenzhen Introduced “AI Civil Servants”