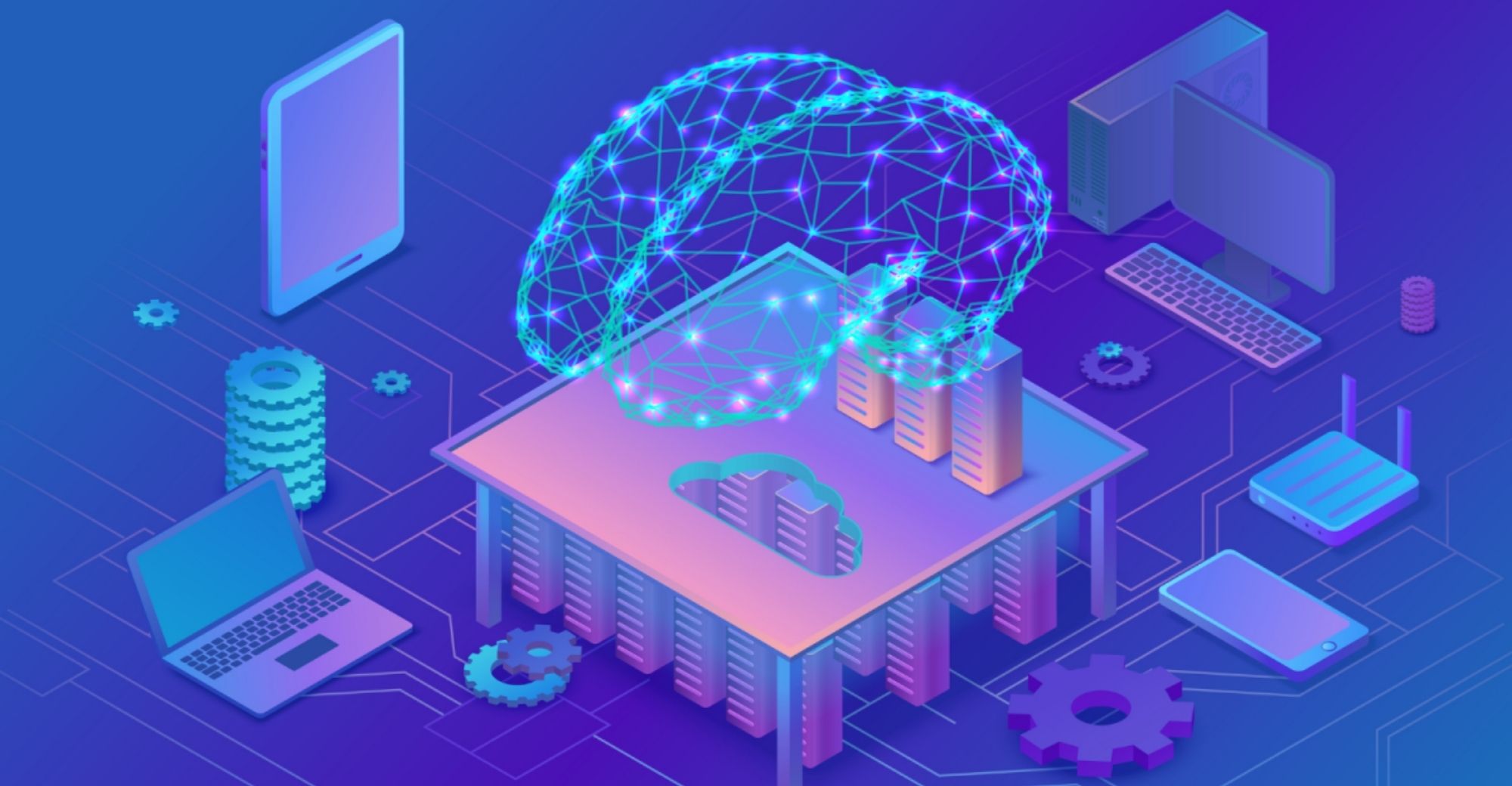
Follow-Up On OpenAI, China's o1-Class Reasoning Models Are Being Introduced One After Another
After OpenAI revolutionized the model training paradigm with the o1 model in September last year, major model companies in China began to fulfill industry expectations at that time, forming a certain scale of follow-up trend for o1-class models.
In the first month of 2025, domestic o1-class models began to be updated intensively. The publishers include Moonshot AI, StepFun, and DeepSeek, which is independent of the entrepreneurial company landscape.
On January 20th, DeepSeek officially released the performance-aligned version of OpenAI-o1, DeepSeek-R1, and simultaneously open-sourced the model weights.
According to the test results disclosed by DeepSeek, it performs comparably to OpenAI-o1-1217 in tasks such as mathematics, coding, natural language reasoning. Particularly excelling with a slight advantage in three test sets: AIME 2024 (American Invitational Mathematics Examination), MATH-500, and SWE-Bench Verified (software development domain).
As a validation of R1's capabilities, several smaller models distilled from the 660B version R1 have been developed. The 32B and 70B models can match up with OpenAI o1-mini in various abilities. These distilled models belong to Qwen series and Llama series. Among them, the performance of the 14B Qwen series distilled model on various inference test sets is significantly better than that of QwQ-32B-Preview.
It should be noted that DeepSeek also open-sourced DeepSeek-R1-Zero which is an achievement that only incorporates RL (reinforcement learning) on top of pre-training without undergoing SFT (supervised fine-tuning).
Due to no human-supervised data intervention, R1-Zero may exhibit poor readability and mixed language phenomena in generation but is still comparable to OpenAI-o1-0912. Its more significant significance lies in focusing on exploring the technical possibility of training large language models solely through reinforcement learning for reasoning ability without supervised fine-tuning provides an important foundation for related subsequent research.
In terms of pricing strategy, DeepSeek continues its identity label as "Pinduoduo" in AI large model field. The pricing for DeepSeek-R1 API services are set at 1 yuan per million input tokens (cache hit) / 4 yuan per million input tokens (cache miss), and 16 yuan per million output tokens. In this price range, the cost for cache-hit input token is less than 2% compared to OpenAI o1 while cache-missed input price and output price are only about 3.6% compared to o1.
Another reasoning model that stands in stark contrast to DeepSeek-R1 is the K1.5 released by Moonshot AI on the same day.
SEE ALSO: Kimi k1.5: The First Non-OpenAI Model to Match Full-Powered O1 Performance
Since November last year, Moonshot AI has updated the k0-math mathematical model, k1 visual thinking model, and other reinforcement learning-based k-series models. K1.5 advances along a multimodal thinking approach and is a multimodal thinking model.
Moonshot AI positions K1.5 as 'multimodal o1.' In simple terms, K1.5 simultaneously possesses both multimodal general capabilities and reasoning abilities.
According to official data, its Short-CoT (Short Consideration) mode's mathematical, coding, visual multimodal general capabilities are comparable to GPT-4o and Claude 3.5 Sonnet; while its Long-CoT (Long Consideration) mode's mathematical, coding, and multimodal reasoning abilities reach the level of OpenAI o1 formal version.
Regarding the training methods of R1 and K1.5, both utilize reinforcement learning, multi-stage training processes, thought chains, and reward models. Based on publicly available information it appears that they have their own respective technical strategies at different stages.
DeepSeek utilized thousands of long CoT cold start data, first fine-tuning the basic model DeepSeek-V3-Base. Subsequently, a large-scale RL training oriented towards reasoning was conducted, and language consistency rewards were introduced to overcome language confusion issues. After supervised fine-tuning (SFT), reinforcement learning applicable to all scenarios was carried out, using different reward rules for reasoning data and general data.
In addition, R1 incorporated the Group Relative Policy Optimization algorithm (GRPO) into reinforcement learning. In terms of effectiveness, it can optimize policies, improve sample efficiency, and enhance algorithm stability.
On one hand, k1.5 expanded the context window of reinforcement learning to 128k; on the other hand, it used a variant of online mirror descent for robust policy optimization. The combination allowed k1.5 to establish a relatively concise reinforcement learning framework that could ensure performance without incorporating more complex techniques such as Monte Carlo tree search, value functions, and procedural reward models.
It is worth noting that k1.5 also introduced 'length penalty' in reinforcement learning to suppress response length by establishing a formula that allocates reward values based on response length and determinism. Additionally, it adopted methods like 'shortest refusal adoption' (selecting the shortest correct response for supervised fine-tuning) to suppress response length.
Another feature of k1.5 is joint training on text and visual data which gives it multimodal capabilities. However Kimi acknowledges that due to some inputs primarily supporting text formats its ability in understanding geometric questions with graphics is not strong enough.
Prior to this, StepFun also launched the experimental version of Step Reasoner mini (referred to as "Step R-mini") on January 16. This is also a reasoning model with ultra-long inference capabilities.
However, it is not fully prepared yet. Currently, in the test set, it mainly benchmarks against OpenAI o1-preview and o1-mini, rather than the complete o1 version. Of course, this should also be related to the model size and training method. In terms of domestic benchmark models, its performance is similar to QwQ-32B-Preview.
Nevertheless, StepFun emphasizes its "balance between arts and sciences," using On-Policy reinforcement learning algorithm. While ensuring mathematical, coding, and logical reasoning abilities, it can also accomplish tasks such as literary content creation and daily chatting.
Since last September when OpenAI revolutionized model training paradigms with the o1 model transformation, major large-scale model companies have begun fulfilling industry expectations at that time. This has led to a certain scale of domestic follow-up trend for o1-class models.
But while all players closely followed o1, OpenAI also unveiled o3 and o3-mini in the release season last December. Although not officially launched yet, based on the data disclosed by OpenAI, o3's performance has significantly improved compared to o1.
For example, in the SWE-Bench Verified software development test set, o3 scored 71.7%, while o1 only had 48.9%; in the AIME2024 test set, o3 achieved an accuracy of 96.7%, compared to 83.3% for o1. Some of o3's performances have begun to show preliminary features of AGI (Artificial General Intelligence).
Of course, o3 also has its own issues. On one hand, the o series models are generally better at tasks with clear boundaries and well-defined definitions but still lack handling capabilities for some real-world engineering tasks. On the other hand, recently in a mathematical benchmark test called FrontierMath, there were doubts about o3's ability due to receiving early access to actual questions through funding from related institutions.
However, the common problem facing large model companies domestically is still clear. From a technical perspective, whether it is DeepSeek-R1 or k1.5 models have not successfully incorporated more complex techniques such as process reward models and Monte Carlo tree search yet; whether these are key methods for further enhancing reasoning abilities remains unknown.
Furthermore, from o1 to o3 with only a three-month interval announced by OpenAI indicates that reinforcement learning brings about scaling up of reasoning stages at a faster pace than GPT series models' pre-training paradigm which operates on an annual basis.
This is the competitive pressure that domestic large model companies must face together now: OpenAI has not only found a clearer technological path but also possesses sufficient resources for rapid verification and advancement. Currently, breakthrough innovations accelerating efficiency will be more crucial than ever before in China's large model industry.